Impact of hybrid surfaces on the droplet breakup dynamics in microgravity slug flow: A dynamic contact angle analysis
This paper aims to investigate the effects of discontinuous wettability on droplet breakup structures under microgravity conditions using a microchannel wall. The approach we adopt is underpinned by the volume-of-fluid methodology, an efficient technique renowned for its accurate resolution of the fluid interface in a two-phase flow. Furthermore, a modified dynamic contact angle model is employed to precisely predict the shape of the droplet interface at and near the wall. Our comprehensive model considers influential parameters such as slug length and droplet generation frequency, thereby providing crucial insights into their impact on the two-phase interface velocity. Validated against existing literature data, our model explores the impact of various configurations of discontinuous wettability on breakup morphology. Our findings highlight the significance of employing a dynamic contact angle methodology for making accurate predictions of droplet shape, which is influenced by the wall contact angle. Emphasis is placed particularly on the effects of slug length and droplet generation frequency.
Advanced Polymer Simulation and Processing – Volume I & Volume II
Polymer-processing techniques are of the utmost importance for producing polymeric parts. They must produce parts with the desired qualities, which are usually related to mechanical performance, dimensional conformity, and appearance. Aiming to maximize the overall efficiency of the polymer-processing techniques, advanced modeling codes along with experimental measurements are needed to simulate and optimize the processes. Our objective with this reprint is to provide a book that exploits the digital transformation in polymer industry, both through the creation of more robust and accurate modeling tools and the development of cutting-edge experimental techniques.
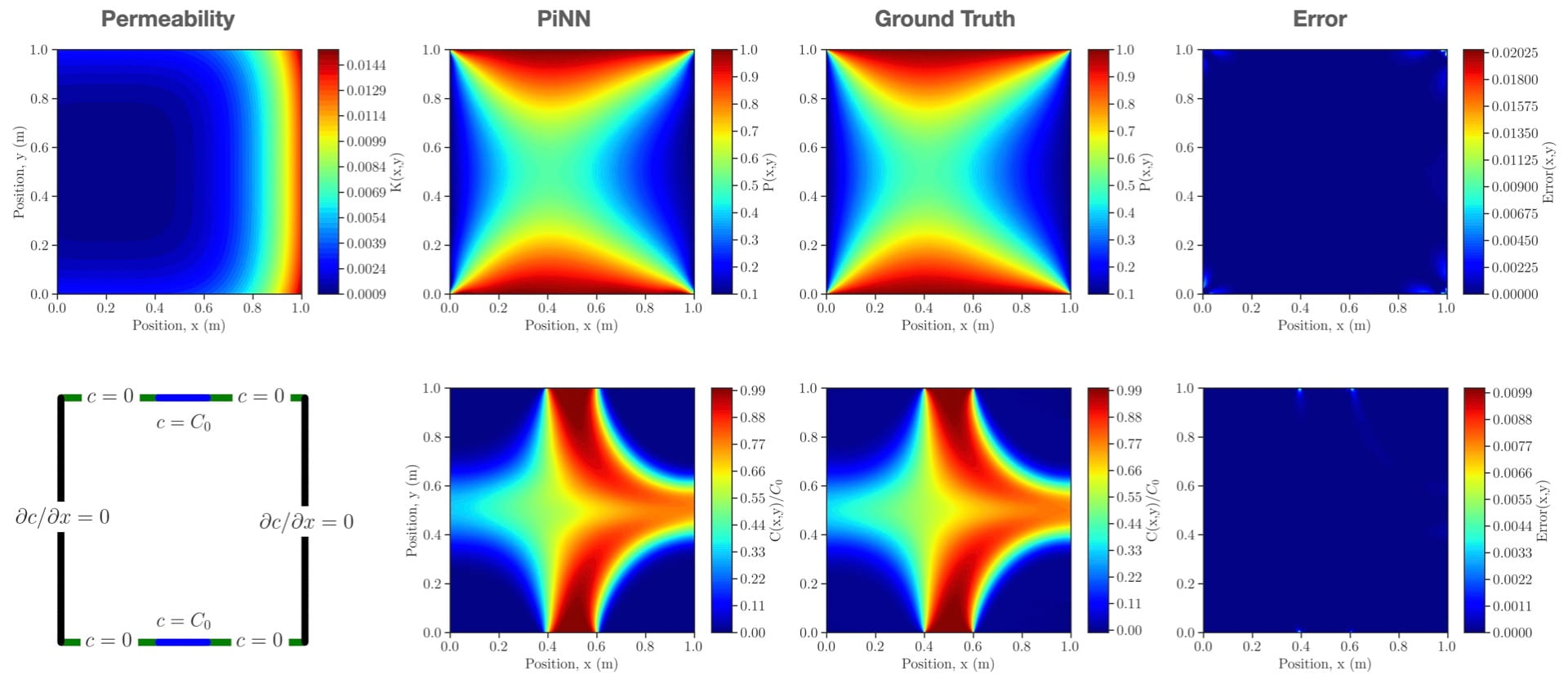
Physics-informed Neural Network (PiNN) to Model Flow in Heterogeneous Porous Media
In scientific computing, physical phenomena are often described using a strong mathematical form consisting of governing differential equations as well as initial and boundary conditions. At each point inside a domain, the strong form specifies the constraints that a solution must meet. The governing equations are usually linear or non-linear PDEs. Some of the PDEs are notoriously challenging and time-consuming to solve, e.g., multiphase flow in heterogeneous porous media. We use mesh-free physics-informed neural networks (PiNNs) to solve these problems. PiNNs show a great potential to be used in modeling dynamical systems described by ODEs and/or PDEs, however, they come with several limitations and shortcomings that required further research.
Multiscale systems (subsurface carbon storage or climate systems modeling ) consider a wide range of physical processes spanning a significant range of temporal (e.g., second to centuries) and spatial scales (e.g., nanoscale, microscale and macroscale). However, the coarse resolutions of simulations (due to cost of computation) limit their use at refined or local scales. To resolve this issue, we are working on different uncertainty quantification, dynamical and statistical downscaling methods to transfer the signal from coarse-scale simulations to higher spatiotemporal resolutions.
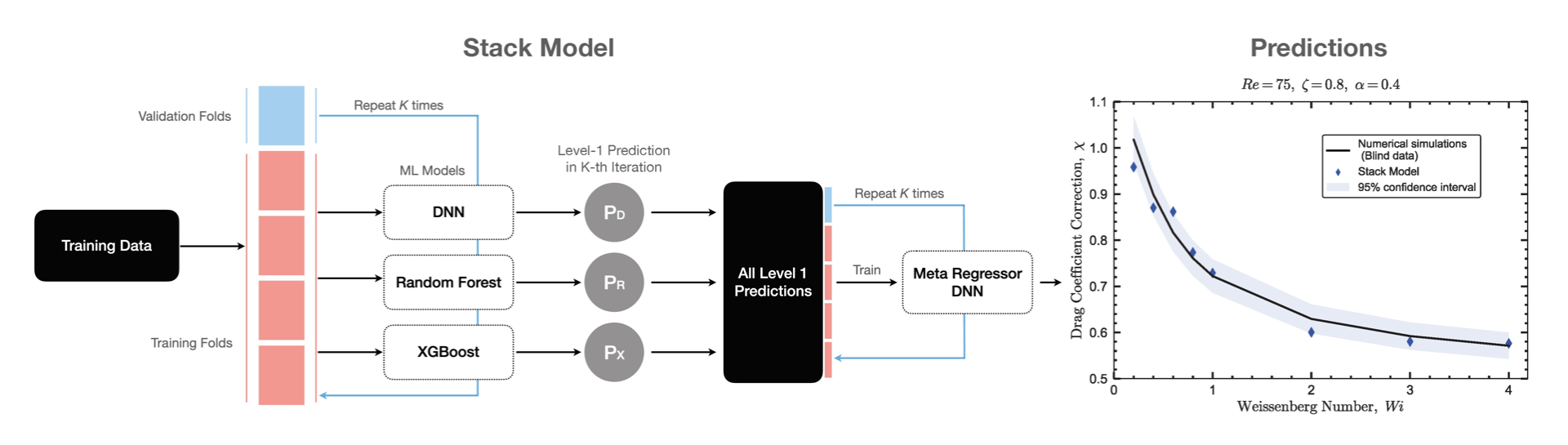
Machine Learning Model to Augment Particle-laden Viscoelastic Fluid Flow Simulations
The current numerical models to simulate complex fluids are slow due to the presence of particles, interplay of multiple physical variables, and flow features occurring at different spatial and temporal scales, i.e., a multidimensional problem. An accelerated computational model can play a major role in exploring the entire parameter space. For this purpose, forward modeling techniques can be augmented with machine learning models that replace bottleneck steps in the simulation procedures. This study provides an example where a meta-model for the viscoelastic drag coefficient of particles is developed to immensely accelerate Eulerian-Lagrangian algorithms commonly applied to model complex fluids.
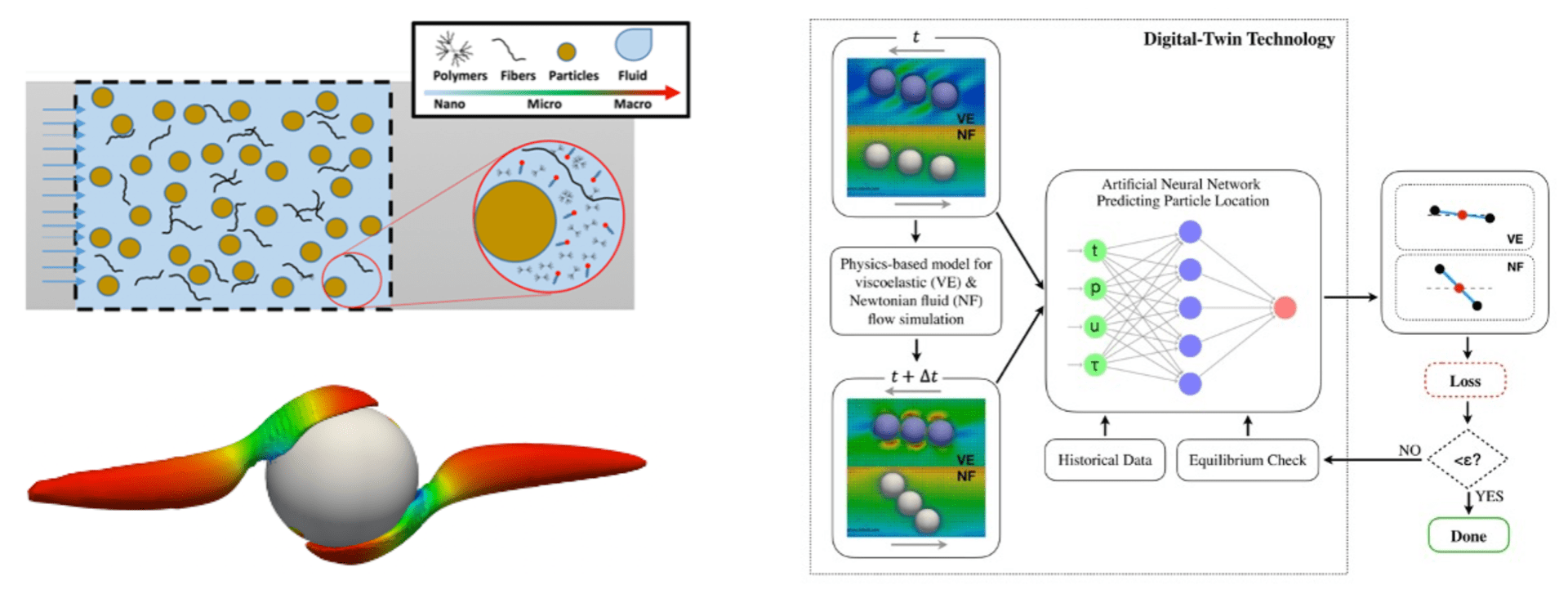
Digital-Twin Driven Model to Simulate Complex Fluids
This project aims to solve one of the main challenge for the application of Deep Learning in the field of complex fluids, which is the scarcity of dataset. We tackle this problem by an in-line integration of traditional computational methods (FV-IBM-DEM) and DL-based modeling, known as a digital-twin technique. These reduced order models will be coupled with the fluid flow solver, and replace computationally expensive methods (i.e., immersed boundary and discrete element methods).
Real-time Hydrogen Leakage Detection and Mitigation
This project aims to provide a breakthrough ML-based detection system powered by integrated sensors running on fifth generation (5G) wireless technology. The 5G wireless technology has features that will not only support high-speed and secure communication but also make big data modeling a lot more efficient on the edge. The 5G has a higher band throughput, is at least 100 times faster than the current network, and reduces signaling overhead significantly providing a 90% reduction in power consumption (i.e., guaranteeing up to 10 years of battery life in low-powered sensor devices). All these features, among many others, make our 5G-based sensing system adaptable to any external and harsh environments, unlike its predecessors that have limited flexibilities. Particularly, this technology can be used to provide extra sensitive characterization and monitoring of hydro-biogeochemical processes in real-time. For hydrogen sensing and leakage detection in remote locations that often have little to no power available, the 5G-based sensing technology can offer a low-cost field deployment while providing accurate integrated surface and/or remote sensor arrays.
Near- and Far-Field Sensor Fusion with Hierarchical Deep Learning
This project aims to provide a technology for improved subsurface characterization, visualization, and diagnostics using sensor fusion. This method will be a major breakthrough in hydraulic fracture technology as enables the reconstruction of multiple signals from thin hydraulic fracture networks that have been stimulated at reservoir scale. In this method, the multimodal datasets typically collected using near and far-field reservoir monitoring tools are fused together to collectively maximize the signal-to-noise ratio and construct an accurate 3D ground truth. This is done through advanced deep learning modeling.